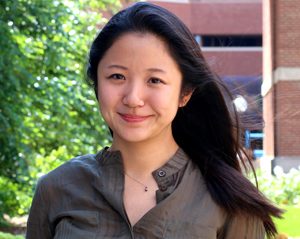
Influenza affects up to 35 million people each year and its seasonal severity can be difficult to predict. To help make forecasts easier, the Centers for Disease Control (CDC) recently held its annual influenza forecast competition where a statistical model from the School of Public Health placed fourth and demonstrated its significant prediction power.
“The great thing about the forecast competition is that it improves forecasting science by trialling and comparing multiple modeling methods from different disciplines, such as computer science and mathematics, which ultimately benefits public health,” says the model’s creator, Environmental Health Science PhD student Yang Liu.
During the project, Liu was mentored by Assistant Professor Matteo Convertino. She also received advice from fellow environmental health doctoral students Joseph Servadio and Maria Sundaram.
Liu developed the model using real-time environmental and public health data to forecast events like the onset of the flu season and its weekly severity across the United States. The model not only finished fourth in overall performance out of twenty-one teams, it also proved to be the best at predicting the seasonal peak timing and short-term outlooks in four out of ten regions. (Miss Liu noted that the model has the potential to accurately predict the spread of other environmentally sensitive infectious diseases, such as meningitis.)
An added aspect of the competition is the CDC put the predictions into practice by sharing the forecasts with its own scientists and on a publicly accessible website.
“The results of these forecasts can be used to inform local public health practitioners and medical service providers regarding the potential pressures they face in the health care system,” says Liu. “At any time during the season, laypeople, public health practitioners, modelers — anyone — can get access to the central repository to explore the visualized forecast results regarding to what is happening around them.”