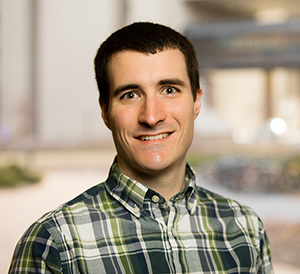
Calculations like health scores and risk factors are essential and common tools for predicting and protecting people from illness or injury. Those numbers are typically created using formulas based on key variables that experts judge to matter most in the development of a score. But some researchers argue that the approach is too subjective and doesn’t take advantage of all the interrelated data now available through systems like electronic health records.
“What if we could pull in all the possible factors that could be related to, for example, heart disease, and use a data-driven method to sift through and pull out the most important ones,” says Joseph Servadio, a PhD student in School of Public Health.
Servadio and his adviser, Adjunct Professor Matteo Convertino, developed an approach, called Optimal Information Networks, to identify the factors and recently demonstrated its use in a paper published in the journal Science Advances.
For the project, the researchers collaborated with Sustainable Healthy Cities and used the method to figure out which pieces of health outcome data are most important in ranking the health of U.S. cities.
The method determines the critical pieces of data by measuring what’s called the “transfer entropy” between them.
“It’s a measure of how much one variable explains another,” says Servadio. “If one variable explains a lot of other variables, you can focus on that piece and ignore the others.”
For example, when the researchers were trying to determine the most important indicators of city health, they looked at HIV/AIDS statistics. In particular, they examined three data points: the rates of HIV-related mortality, HIV diagnosis, and AIDS diagnosis. The Optimal Information Networks method showed that HIV mortality is a more influential aspect of city health and HIV/AIDS diagnosis rates could be ignored because they are components of the death rate.
Servadio said the method could be of tremendous and wide value to researchers, policymakers, and health care providers.
“Our method can be used by anybody who wants to do variable reduction,” says Servadio. “If you want to use a large set of variables to make a calculation and figure out what subset is going to be most valuable, you can use our method.”