Many experts talk about the potential of artificial intelligence (AI) and machine learning to fundamentally improve disease research and overall health outcomes, but few people know that these technologies are already employed in more mundane, administrative parts of the health care system. Many hospitals, for example, employ AI-assisted predictive models to help them perform a range of tasks, including automating billing procedures and appointment scheduling.
Although use of these AI-assisted predictive tools has increased in hospitals across the U.S., effective evaluation of them is lacking. In addition, previous work has found that these kinds of models can exhibit bias, which means they do not function properly for all patients. Until now, it has been unclear whether hospitals are evaluating the models they use for bias.
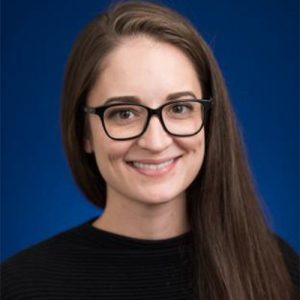
A new study from the University of Minnesota School of Public Health (SPH) offers one of the first national analyses of how hospitals in the U.S. are using AI-assisted predictive models, and also evaluates how hospitals are using them.
By analyzing responses from 2,425 acute-care hospitals that participated in the 2023 American Hospital Association (AHA) Annual Survey, the study explores differences in adoption, use, and evaluation capacity among hospitals — and sheds light on a growing digital divide between hospitals with greater financial resources and under-resourced hospitals.
The study, published in Health Affairs, found:
- Use of predictive models is widespread. Approximately 65% of U.S. hospitals reported using AI-assisted predictive models. These models were most commonly employed to predict inpatient health trajectories (92%), identify high-risk outpatients (79%), and facilitate scheduling (51%).
- Less than half of hospitals evaluated the bias in their models. While 61% of hospitals evaluated their predictive models for accuracy, only 44% conducted similar evaluations for bias. Better-funded hospitals that developed their own models were more likely to conduct evaluations for both accuracy and bias compared to those using models from external developers.
- Evidence of a potential digital divide. Hospitals with more financial resources and technical expertise were more likely to have and evaluate AI models than under-resourced hospitals.
“By focusing on the differences in evaluation capacity among hospitals, this research highlights the risks of a growing digital divide between hospital types, which threatens equitable treatment and patient safety,” says Paige Nong, SPH assistant professor and lead author. “Many better-funded hospitals and academic medical centers can design their own models tailored to their own patients, then conduct in-house evaluations of them. In contrast, critical-access hospitals, rural hospitals, and other hospitals with fewer resources are buying these products ‘off the shelf,’ which can mean they’re designed for a patient population that may look very different from their actual patients and may not reflect the needs of local patient populations.
“Policymakers should address this challenge and ensure that all hospitals have the capacity to evaluate the AI models they are using.”
The study emphasized the need for policies and practices to promote fair and accurate AI, including financial incentives, technical support, and enhanced regulatory oversight.
Watch
Paige Nong discusses her research, which focuses on how healthcare organizations are using artificial intelligence and whether these AI tools are being used equitably across healthcare organizations.