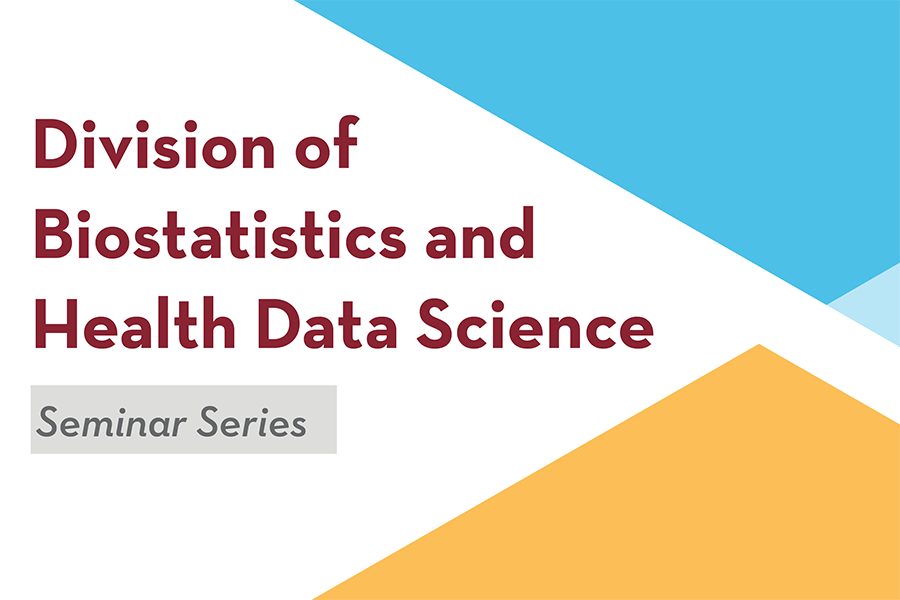
BHDS Plan B Presentation with Julia Kancans
Monday, July 29 @ 3:00 pm - 4:00 pm CDT
Monday, July 29 @ 3:00 pm - 4:00 pm CDT
Assessing Poisson-distributed Differentially Private Synthetic Data Using County-Level Data from Minnesota on the 1980 Leading Causes of Death
Presented by Julia Kancans
Masters Candidate in Biostatistics
Plan B Adviser: Harrison Quick
CDC WONDER is a database with useful public health information that can be stratified by a number of demographic factors. However, the database is susceptible to targeted attacks and (post-1989) suppresses counts of 1-9. As an alternative to releasing data with suppression, releasing synthetic data has been proposed as a potential method for preserving both individuals’ privacy and the utility of data. Specifically, differentially private Poisson-distributed synthetic data with prior predictive truncation has been proposed as a mechanism for generating synthetic data with provable privacy protections (as measured by a privacy budget). This method has been evaluated on datasets consisting of county-level heart disease and cancer deaths in Pennsylvania and shown to preserve both racial and urban/rural disparities but has yet to be evaluated in acute disease mortality or causes of death with smaller age-standardized mortality rates. Here, we explore the viability of using this approach to generate synthetic data for several leading causes of death using county-level data from Minnesota, with a focus on the synthetic data’s ability to preserve urban/rural disparities in the cause-specific death rates. In addition to highlighting the performance of the approach, we also provide commentary on how to select the level of the privacy budget.
We strive to host inclusive and accessible events. To request accommodations or additional information, please contact biostats@umn.edu