Adam Kaplan
PhD, Biostatistics
Co-Authors:
Thomas A. Murray
Advisor:
Dr. Thomas A. Murray
Educational Objectives:
- Evaluate the use of strong external information in Bayesian Hierarchical modeling of complex problems.
- Identify key issues within the practice of teaching statistics or data visualization that can be improved upon for student absorption in K-12, undergraduate, and professional degrees.
- Design a workshop class for data cleaning
Keywords:
Adaptive Design, Personalized Medicine
Abstract
Recently, spinal epidural neurostimulation is being considered for rehabilitation of persons suffering from partial spinal cord injury. The neurostimulator must be programmed by a neurosurgeon, yet little work has been done to develop rigorous methods for optimally programming the device. We propose an adaptive design to efficiently optimize programming of the neurostimulator based on monthly evaluations of patient reported preferences. Preferences for the eligible device configurations are estimated after each month through a conditionally auto-regressive model that assumes preference for one configuration is related to preferences for neighboring configurations. Using the adaptively updated preferences, a group of configurations is programmed into the device for the patient to evaluate during the next month. This selection is based on a balance of device exploration and preference maximization. We repeat this process until a specified stopping rule or the trial-end is reached. We show simulation studies to evaluate the overall quality of the adaptive trial for various configuration selection strategies and the effects of stopping the trial early.
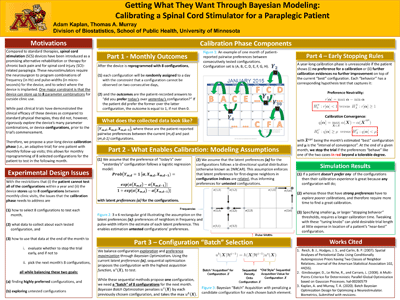
View Poster (PDF)