Sarah Samorodnitsky
PhD, Biostatistics
Co-Authors:
Katie Hoadley, Eric Lock
Advisor:
Dr. Eric Lock
Educational Objectives:
Demonstrate the application of a Bayesian hierarchical model in the setting of cancer patient survival based on somatic mutations. Compare the effect of these mutations on cancer survival based on cancer type.
Keywords:
Cancer Survival, Genomic Mutations
Abstract
We built a novel Bayesian hierarchical survival model based on the somatic mutation profile of patients across 50 genes and 27 cancer types. The pan-cancer quality allows for the model to “borrow” information across cancer types, motivated by the assumption that similar mutation profiles may have similar (but not necessarily identical) effects on survival across different tissues of origin or tumor types. The effect of a mutation at each gene was allowed to vary by cancer type, whereas the mean effect of each gene was shared across cancers.
Within this framework, we considered 4 parametric survival models (normal, log-normal, exponential, and Weibull), and we compared their performance via a cross-validation approach in which we fit each model on training data and estimate the log-posterior predictive likelihood on test data. The log-normal model gave the best fit, and we investigated the partial effect of each gene on survival via a forward selection procedure.
Through this we determined that mutations at TP53 and FAT4 were together the most useful for predicting patient survival. We validated the model via simulation to ensure that our algorithm for posterior computation gave nominal coverage rates. View the code used for this analysis and the summary of results.
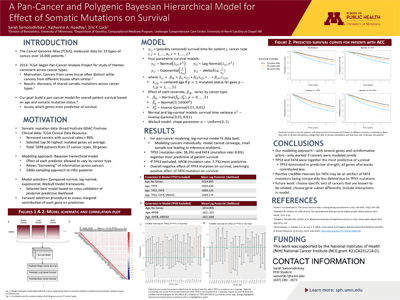
View Poster (PDF)