Recent technological advances have enabled the production of vast amounts of data types that can help health researchers better understand complex diseases, such as cancer, cardiovascular diseases and neurodegenerative disorders. Called “multi-omics data” and encompassing genomics, epigenomics, proteomics and transcriptomics, these types of data provide a vast and holistic view of the biological systems of diseases, helping researchers unravel the underlying biological mechanisms of diseases and improve clinical outcomes. However, the sheer volume and diversity of this data makes it challenging for health researchers to identify important biomarkers among hundreds of thousands of data points.
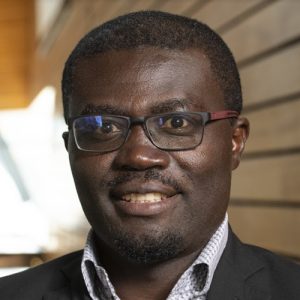
A new School of Public Health (SPH) study will directly address this challenge by developing and applying Bayesian statistical learning methods that will help researchers analyze vast amounts of multi-omics data. Bayesian methods organize data by assigning probabilities to events or parameters in data sets based on experience or other factors. A key advantage of Bayesian models is their ability to handle non-linearity, an essential feature when modeling disease and other biological systems. The SPH researchers will use these methods to identify:
- Key predictive pathways and their corresponding important molecules, such as genes, proteins, metabolites and lipids.
- Clinically meaningful molecular disease subtypes; and
- Predictive and prognostic biomarkers that contribute to the joint association (or regulatory networks) between omics data types. Omics data will be put into a Bayesian predictive statistical model for the purpose of selecting omics features that are associated with disease outcomes.
“By applying the proposed method to publicly available datasets such as the Cancer Genome Atlas, dbGAP, and Genotype-Tissue Expression, and to non-public datasets obtained from our collaborators, these models hold great promise for advancing our understanding of complex diseases,” says Thierry Chekouo, SPH assistant professor and lead researcher on the study. “We plan to develop robust, computationally efficient and user-friendly software free of charge for the application of our methods, and make it available to the community of scientists, data scientists, biostatisticians and others who can use it to advance their research into complex diseases.”
While the study is expected to be completed in five years, Chekouo says he expects to have preliminary findings by the end of 2024. Results will be disseminated in multiple ways. Freely available software for the proposed methods will be available online. Details of the methods will be published in peer-reviewed, statistical methods journals.
The research is supported by the National Institute Of General Medical Sciences of the National Institutes of Health.